Calling for Rigorous Method Comparison in Machine Learning for Drug Discovery
Machine learning (ML) is revolutionizing drug discovery by providing in silico surrogates for expensive and time-consuming experiments. But the field is facing a replicability crisis, with many studies failing to reproduce results. This lack of rigor is slowing the adoption of ML in drug discovery.
In my blog, a post titled A few points about AI model validation already addressed this issue, but that piece only shared brief thoughts and fell far short of being a comprehensive guide on the topic. In this regard, this paper on ChemRxiv, titled “Practically significant method comparison protocols for machine learning in small molecule drug discovery”, can be considered a valuable guide that can greatly assist everyone from those directly creating predictive models using AI/ML technologies to researchers who want to choose the model that best fits their needs. Before discussing the content of the paper, looking at which institutions the authors belong to on the first page will make you reflect once more on the value of this paper.
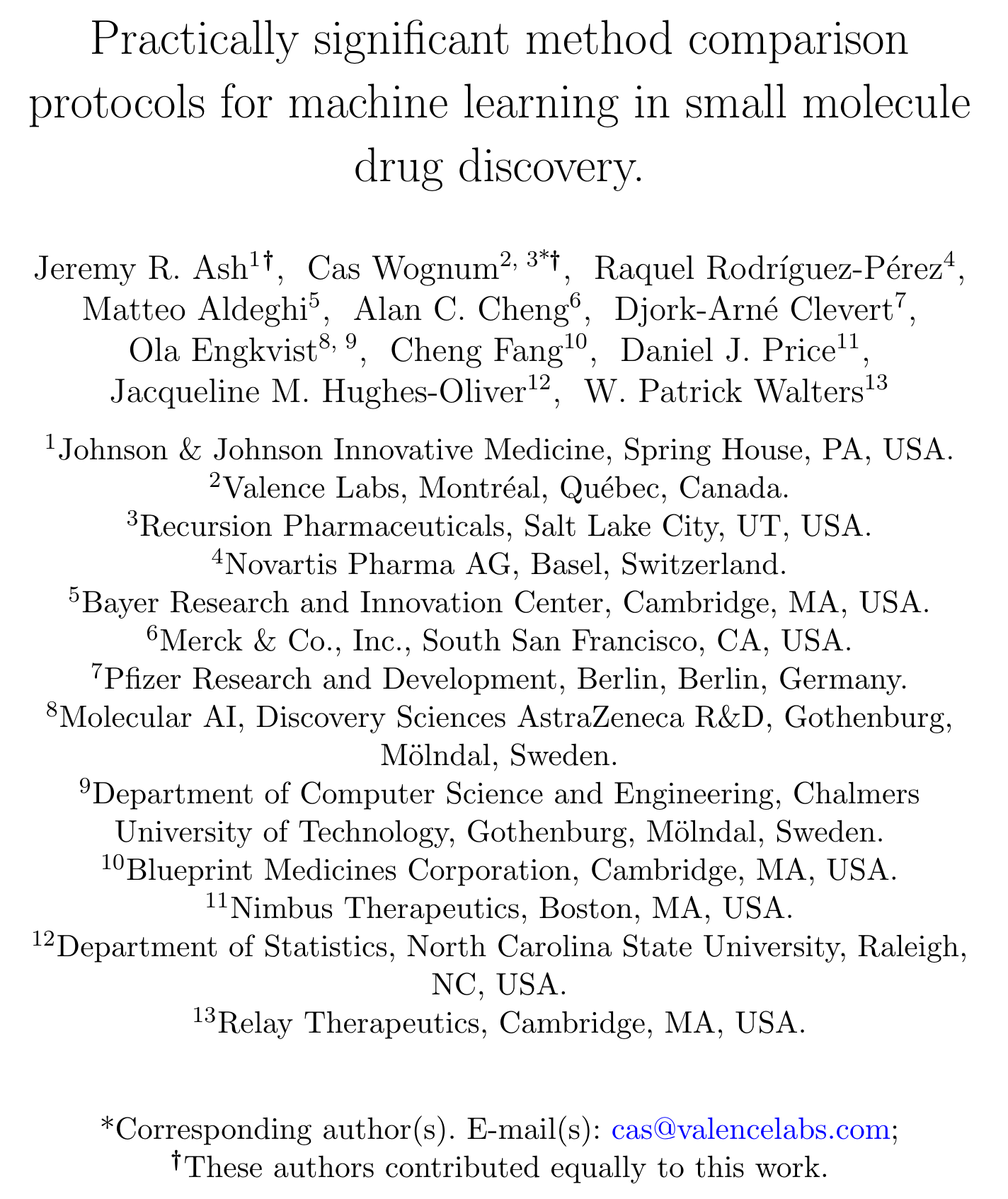
I would say no matter how much I organize the content, readers will ultimately need to read and study the paper themselves. Those who write papers should certainly refer to the suggestions presented here, but even those who think they can simply read and use papers about such models should keep this in mind. Otherwise, they may end up believing claims in papers that lack solid evidence and make incorrect judgments, which can ultimately negatively impact their work.
Think of it this way: you’re trying to find the best tool to predict how a new drug will behave in the body. But there are lots of tools out there, each claiming to be the best. How do you know which one to trust? Trusting the results of a paper that lacks solid evidence and proceeding with one’s work or research based on that can lead to failures, which is likely to foster an unfounded distrust in the technology. This, in turn, reduces the chances of embracing valuable technology and contributing to success.
This paper provides essential guidelines for those evaluating ML methods for predicting properties of molecules (like solubility or how a drug might bind to its target).
Here’s what you should keep in mind:
1. Don’t rely on just one test: It’s like trying to figure out if a coin is fair by flipping it just once. You need to test things repeatedly to be sure. The authors suggest using a technique called “repeated cross-validation” to get a more reliable estimate of how well a method will perform.
2. Beyond just “better”: It’s not enough to just say one method is better than another. You need to understand how much better it is. This is called “practical significance” and means the difference is big enough to actually make a difference in your drug discovery work.
3. Visualize and communicate: Don’t just show a bunch of numbers. Use graphs and plots to help visualize the differences between methods. And make sure you explain your findings in a way that everyone on your team can understand.
By following these guidelines, you can make more informed decisions about which ML methods to use in your drug discovery research.
Comments